New NITC Dissertation Will Improve the Quality of Freeway Volume Data
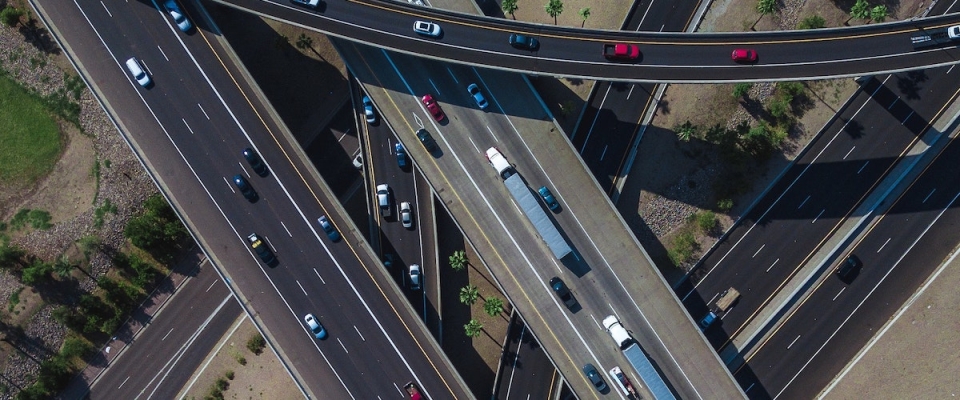
The National Institute for Transportation and Communities (NITC) is proud to introduce a new Dissertation Fellow, Adrian Cottam of the University of Arizona. Cottam's doctoral research project, Machine Learning and Big Data-Based Approaches for Quality Freeway Volumes, will focus on improving the quality of freeway volumes and expanding their spatial availability.
"I was drawn to Transportation Engineering because it is a field where you can make major impacts to better your community. I found that by merging my passion for Computer Science with Transportation Engineering, I could use data to obtain more information on how local transportation could be improved through Intelligent Transportation Systems (ITS) and performance measurement. Throughout this process, I found that it was frequently a challenge obtaining high-quality data, or having access to data where you need it. This drove me to focus my dissertation on using machine learning techniques to improve data quality, and estimate traffic parameters where sensors were unavailable. My research is specifically focused on freeways, and seeks to use available data sources, such as crowdsourced data, to estimate data that isn’t always available. I am a firm believer in applied research, and hope to better my community through my research efforts. As such, I seek to develop methods that can be applied in a practical way, so that local transportation engineers can have better data to make more informed decisions for Arizona roadways," Cottam said.
Traffic volumes are essential for the operation of Intelligent Transportation Systems (ITS) and for use in performance metrics for Transportation Systems Management and Operations (TSMO). Maintaining high quality freeway volumes is paramount to ensure that freeway ITS solutions, such as ramp meters, operate smoothly. Furthermore, freeway performance metrics need quality volume data to provide an accurate portrayal of real-world conditions. Cottam's dissertation has three components:
- Using loop detector data and crowdsourced data to develop a machine learning agent network to estimate volume for faulty loop detectors;
- Using crowdsourced data and recurrent neural networks to estimate freeway volumes at locations where no loop detectors are available;
- Using crowdsourced data to estimate freeway volumes at locations where no loop detectors are available for several different types of models using spatial embedding.
These methods can provide benefit for transportation professionals, as machine-learning volume data estimation can allow them more time to repair faulty detectors, as well as reducing the need for freeway sensors in some areas.
Adrian Cottam, University of Arizona
Adrian Cottam is a PhD Candidate in transportation engineering and data science at the University of Arizona (UA) and the IT Manager for UA's Center for Applied Transportation Sciences (CATS). He earned his master's in transportation engineering from UA in 2019, and during that time he worked as a graduate research assistant in the Smart Transportation Lab with NITC researcher Yao-Jan Wu. Adrian also served as an officer in the Institute of Transportation Engineers (ITE) University of Arizona student chapter. Cottam is an Eisenhower fellow as well as a GAANN fellow, and expects to complete his PhD program in 2023. Connect with Adrian on LinkedIn.The National Institute for Transportation and Communities (NITC) is one of seven U.S. Department of Transportation national university transportation centers. NITC is a program of the Transportation Research and Education Center (TREC) at Portland State University. This PSU-led research partnership also includes the Oregon Institute of Technology, University of Arizona, University of Oregon, University of Texas at Arlington and University of Utah. We pursue our theme — improving mobility of people and goods to build strong communities — through research, education and technology transfer.
Photo by Jared Murray on Unsplash