New Radar Sensor Technology for Intelligent Multimodal Traffic Monitoring at Intersections
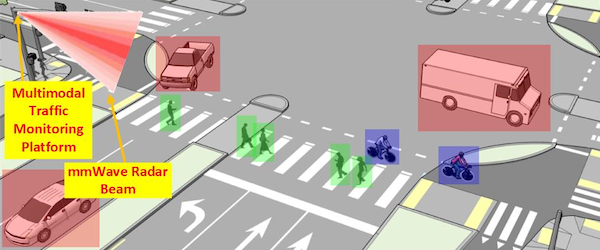
Intelligent Transportation Systems (ITS) need traffic data to run smoothly. At intersections, where there is the greatest potential for conflicts between road users, being able to reliably and intelligently monitor the different modes of traffic is crucial.
The Federal Highway Administration (FHWA) estimates that more than 50 percent of the combined total of fatal and injury crashes occur at or near intersections. For pedestrians the intersection is a particularly dangerous place: the City of Portland, Oregon identified that two-thirds of all crashes involving a pedestrian happen at intersections. And when darkness comes earlier in fall and winter, crashes increase dramatically. So knowing what's going on in low-visibility conditions is essential for mobility and safety of all road users.
Some agencies use cameras to monitor traffic modes, but cameras are limited in rainy, dark or foggy conditions. Some cities use radar instead of cameras, which works better in low-visibility but typically can't provide as rich a picture of what's going on. Conventional radar gives movement and position data for all approaching entities, but it's very hard to tell the difference between modes with any reliability.
In the latest report funded by the National Institute for Transportation and Communities (NITC), Development of Intelligent Multimodal Traffic Monitoring using Radar Sensor at Intersections, researchers Siyang Cao, Yao-jan Wu, Feng Jin and Xiaofeng Li of the University of Arizona have tackled the issue by developing a high-resolution radar sensor that can reliably distinguish between cars and pedestrians. This sensor also supplies the counts, speed, and direction of each moving target, no matter what the lighting and weather are like. In the future, they plan to further refine their model to interpret more complex data and be able to identify additional modes. Hear more from Dr. Siyang Cao in this research recap video:
WHY USE mmWAVE RADAR?
The prototype device used a high-resolution millimeter-wave (mmWave) radar sensor that outperforms cameras in low-visibility conditions and beats conventional radar by providing a richer picture.
"The mmWave radar is also different from other sensors in that it can provide relatively stable radial velocity, which is very helpful for us to identify the speed of vehicles," Cao said.
This gives the system an advantage over light-based sensors like LiDAR. LiDAR systems are able to "see" in great detail, making it easy to determine what an object is; but they have difficulty with movement and speed. MmWave radar can resolve the speed of a moving target much more reliably than LiDAR.
"The key problem in multimodal traffic monitoring is finding the speed and volume of each mode. A sensor must be able to detect, track, classify, and measure the speed of an object, while also being low-cost and low power consumption. With real-time traffic statistics we hope to improve traffic efficiency and also reduce the incidence of crashes," Cao said.
The mmWave radar sensor also works on a lower frequency band than LiDAR, making it more stable under different weather conditions (rain, snow, fog, smoke, etc.). In multimodal traffic monitoring, sensors need to be employed outdoors, so mmWave radar is an optimal choice.
TESTING THE SENSOR AT AN INTERSECTION
The research team developed a multivariate Gaussian mixture model (GMM) to interpret the information from the mmWave radar sensor. First, the sensor obtains a rich radar point cloud representation. Then the model segments the point clouds into three kinds of objects: pedestrian, sedan, and "clutter." Clutter just refers to non-moving surfaces like buildings, trees or other objects. Once this is done, the data stream is transmitted wirelessly to a laptop and displayed as a visual image. In the lab, Cao and his students calibrated the device so that its output would match what a camera sees.
The prototype built by the UA research team is low-cost, low-weight, and of a compact size that's easy to install. The researchers installed their sensor at an intersection in Tucson and set up a laptop in a nearby parking lot to test it. With the GM model they developed, it yielded promising results at both detecting and correctly identifying objects.
Signalized intersections like the test site are critical spots for collecting such mixed-traffic data, because the most conflicts and crash occurrences tend to involve multiple transportation modes.
EXPANDING TECHNOLOGY FOR MULTIMODAL TRAFFIC
"We realize that sensor technology is moving to a stage that's going to have a lot of new applications. On one hand, the cost of sensors is dropping and their performance is improving significantly. Meanwhile the surrounding technology—for example battery technology, communications, and computation enabled by artificial intelligence— is also improving. For multimodal traffic monitoring, a sensor can collect information that can be shared with drivers via a next-generation communication network to improve mobility and safety at intersections," Cao said.
In the future, the researchers hope to further refine the model to be able to identify additional modes such as motorcycles, bikes, trucks and buses. Once refined, this sensor could play a key role in facilitating a reliable and accurate city-wide traffic network. The project's GitHub repository offers more information, including data, video and images from the test deployment.
In addition, the outcomes of this project (the GMM and the prototype) offer useful insights for people developing other advanced technologies to create equitable, healthy, and sustainable smart cities. Through leading in the development and deployment of innovative practices and technologies, this research can help improve the safety and performance of the nation’s transportation system.
Dr. Feng Jin, who worked on this project as a PhD student, graduated from the University of Arizona in 2020 and now works as a senior radar perception engineer at NVIDIA.
ABOUT THE PROJECT
Development of Intelligent Multimodal Traffic Monitoring using Radar Sensor at Intersections
Siyang Cao, University of Arizona; Yao-Jan Wu, University of Arizona
- Download the Final Report (PDF)
- Download the Project Brief (PDF)
- Visit the project GitHub page
- Watch the recorded November 2021 Webinar
- Watch the NITC Research Recap Video
Images by Siyang Cao and Yao-Jan Wu, University of Arizona
This research was funded by the National Institute for Transportation and Communities, with additional support from the University of Arizona and the City of Tucson, Arizona.
RELATED RESEARCH
To learn more about this and other NITC research, sign up for our monthly research newsletter.
- Data-Driven Mobility Strategies for Multimodal Transportation
- Connected Vehicle System Design for Signalized Arterials
- Visual Exploration of Utah Trajectory Data and their Applications in Transportation
The National Institute for Transportation and Communities (NITC) is one of seven U.S. Department of Transportation national university transportation centers. NITC is a program of the Transportation Research and Education Center (TREC) at Portland State University. This PSU-led research partnership also includes the Oregon Institute of Technology, University of Arizona, University of Oregon, University of Texas at Arlington and University of Utah. We pursue our theme — improving mobility of people and goods to build strong communities — through research, education and technology transfer.