NITC Dissertations Explore Perceived Safety, Machine Learning and Crash Data
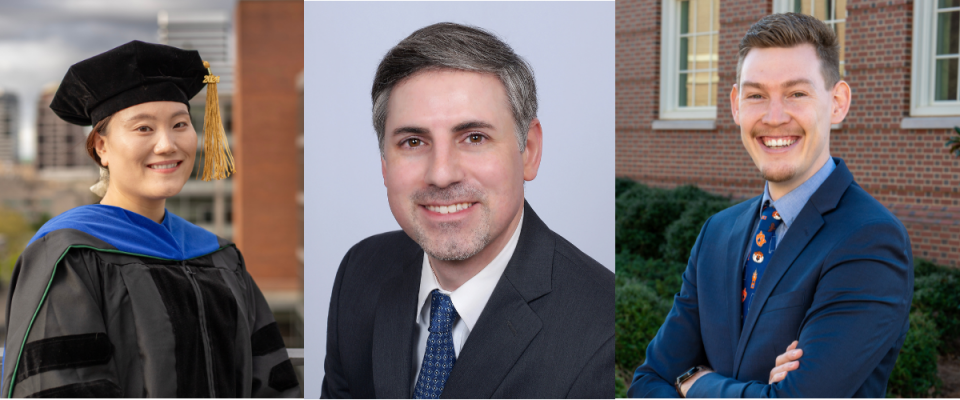
Three dissertations supported by funding from the National Institute for Transportation and Communities (NITC) take on different transportation challenges, exploring ways to improve safety and connectivity in communities. Farzin Maniei, Adrian Cottam, and Kyu Ri Kim all completed their doctoral research with support from the NITC program. Read on to learn more about each of their projects.
Adrian Cottam, University of Arizona
Machine Learning and Big Data-Based Approaches for Quality Freeway Volumes
"If you boil it down, the whole purpose of my dissertation is to take existing resources that DOTs have, and try and make better use of them. For example, the Arizona Department of Transportation has loop detectors, but only in the Phoenix region. So it's a very small area out of the total land mass of Arizona. But they have other datasets, like INRIX and other crowdsourced datasets, throughout the state. So the idea of my dissertation is, can we use some of these more expensive data sets coming from the loop detectors and estimate them with some of these lower-cost and more widely available datasets?"
Traffic volume data is essential for transportation agencies for performance measurement and for implementing intelligent transportation systems (ITS) strategies. However, this data is typically collected by freeway traffic sensors, such as loop detectors. Freeway traffic sensors can be prone to failures due to communication loss or sensor failures. Furthermore, freeway traffic sensors are costly and require maintenance, making them expensive to deploy with wide spatial coverage. To address these issues with freeway traffic sensors, Cottam proposes three methodologies. The first estimates volumes for traffic sensors that have failed, ensuring ITS implementations continue to receive traffic volume data. The second estimates volumes for locations where freeway traffic sensors are unavailable. The final methodology estimates passenger car equivalency (PCE) factors for locations where freeway traffic sensors are unavailable. Two chapters of the dissertation have been published in journals:
- Large-Scale Freeway Traffic Flow Estimation Using Crowdsourced Data: A Case Study in Arizona
- Machine-learning approach for estimating passenger car equivalent factors using crowdsourced data
Kyu Ri Kim, Portland State University
The Central Role of Perceived Safety in Connecting Crash Risk Factors and Walking Behavior
When Kyu Ri Kim was seven years old, she was struck by a car while walking in her neighborhood where there was no separate walkway for pedestrians in Seoul, South Korea.
"That was the real starting point, my personal experience. And I'm curious whether other people really do understand the real risk around them. What are the crash risk factors around them and how are they different from individual perceived risk?" Kim said.
Her dissertation illuminates the relationship between pedestrian crash risk factors and perceived safety, as well as the relationship between safety attitudes and walking behavior. How the interplay between these factors influences people's behavior needs to be better understood in order to improve pedestrian safety and the walking environment. Overall, pedestrians' attitudes were mainly determined by their subjective experiences in a given environment, rather than their actual crash risk.
Farzin Maniei, University of Texas, Arlington
"Knowing that there has not been a day without a fatality on Texas roadway since November 7th, 2000, I have been encouraged to focus my dissertation on traffic safety analysis and traffic crash prediction models to mitigate the socioeconomic burden of traffic crashes," Maniei said.
Both crash frequency analysis and real-time crash prediction models typically divide a highway into segments with a constant length for data aggregation. Despite the significant impact of the segment length, as shown in several previous traffic safety studies, no standard approach exists for determining a recommended segment length for crash data aggregation. Maniei's dissertation aims to establish a methodology for determining a recommended fragment size, which will benefit future crash frequency analysis and crash prediction models. The study attempts to capture various groups of traffic crashes, identify their corresponding hotspots, and understand their associated contributing factors. See also the journal publication:
The National Institute for Transportation and Communities (NITC) is one of seven U.S. Department of Transportation national university transportation centers. NITC is a program of the Transportation Research and Education Center (TREC) at Portland State University. This PSU-led research partnership also includes the Oregon Institute of Technology, University of Arizona, University of Oregon, University of Texas at Arlington and University of Utah. We pursue our theme — improving mobility of people and goods to build strong communities — through research, education and technology transfer.