Report details simpler way to track freight on Oregon's highways
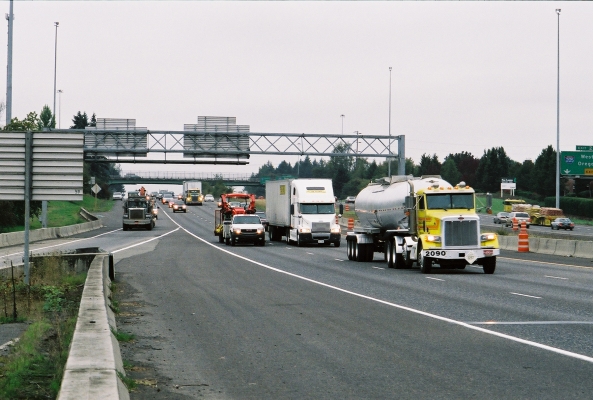
Freight transportation is an important part of Oregon’s economy. Helping the statewide freight-transport system run more efficiently means better understanding the movements of trucks on the highways. By monitoring the progress of individual trucks, the Oregon Department of Transportation can obtain valuable performance metrics such as travel time, travel delays, and origin-destination flows. This information can help identify slow passages or bottlenecks in the highway system.
Tracking individual trucks, however, can be problematic. To follow the movements of a truck on the freeway, typical methods might include putting in automatic vehicle identification (AVI) tags, or acquiring a license-plate-recognition system to be used at checkpoints. For ODOT, this could mean purchasing expensive new equipment. Moreover, these tracking methods can raise privacy concerns.
In an OTREC-sponsored research project, Portland State University’s Chris Monsere looked into alternative methods for obtaining those helpful freight metrics without installing tracking units in every single truck. The details of Phase 2 of the project, which expand upon and further refine the results of Phase 1, can be found here. For more information, download the OTREC final report: Exploratory Methods for Truck Re-identification in a Statewide Network Based on Axle Weight and Axle Spacing Data to Enhance Freight Metrics: Phase 2
Vehicle reidentification is the process of matching vehicles from one point on the roadway to the next. With the process developed by Monsere this can be done anonymously, and inexpensively, using tracking methods that are already in place. Oregon’s highways, like elsewhere in the US, are dotted with weigh-in-motion (WIM) and automatic vehicle classification (AVC) stations. At these stations, vehicle attributes including truck weight, axle spacing and axle weight are recorded.
This information is collected for reasons unrelated to freight efficiency; for example, truck weight data from WIM stations is often used in bridge design, to learn the average weight of vehicles that will be passing over the bridge. Monsere and his research team used archived data to single out trucks passing through one WIM station, and then attempted to find those same trucks in the data from a second WIM station, several hundred miles from the first. Using the axle weight, axle spacing, and truck weight, they matched the records to reidentify the trucks as they passed through the second station.
Since some of the trucks that Monsere and his team tested (about 20-35 percent of them) were carrying Green Light transponders, which also register at the WIM stations, these particular trucks provided the needed data to test how well the reidentification methods were working. It was found that when 95 percent of the trucks passing through the stations were tested, the team was able to match them with 90 percent accuracy. The accuracy can be increased by reducing the total number of trucks matched; for example, matching 40 percent of all trucks crossing both stations results in 98 percent accuracy.
In Phase 1 of the project, the researchers used a Bayesian algorithm to calculate the probability of a match between two vehicles at the upstream and downstream stations. In Phase 2, they explored the possibility of using a neural network model to predict the results. They found that while both methods worked, the Bayesian method was more accurate. During Phase 2 of the project, Monsere and his team also did a comprehensive analysis to determine what factors impacted the accuracy of the results. They found that sensor accuracy and volumes had the greatest impacts on accuracy, whereas the distance between the two data points had less of an impact. They also identified an imperfection in the system: there is no mechanism to account for trucks that enter the highway somewhere between the two checkpoints, passing through the second station without passing through the first. This has the potential to muddy up the results, but even with these limitations, the reidentification system – with a 95% rate of accuracy, on average – works well enough to be put into practice.